27 July 2022
How will AI, ML, cloud services and automation shape the future of asset management? flow reports on a webinar hosted by The Asset magazine that examined how custodians can help investors navigate social and ethical concerns, while continuing to deploy new digital solutions
Automation, artificial intelligence (AI), machine learning (ML) and cloud technologies are fast becoming essential tools for the financial services industry – not least within asset management and securities services. These tools are already being used to enhance a variety of processes, from front-office activities to compliance and operations in the back office. They promise to usher in a new age of competitive services and efficient processing based on digital intelligence.
However, realising the transformational potential of these technologies requires advance planning and preparation. The importance of ensuring that AI, ML and cloud systems are properly governed – based on good knowledge of the underlying technology, rules, ethical standards, and human domain expertise – cannot be overstated. It is only with this proper foundation that market infrastructure participants, from broker-dealers to global custodians, can harness the full value of automation.
To take a closer look at where automation is heading in securities services, four industry experts joined Mathew Kathayanat, Head of Product, APAC at Deutsche Bank, for ‘Unlocking the value of Automation in Asset Management’ the first webinar in the Securities Services APAC leadership series hosted by The Asset.
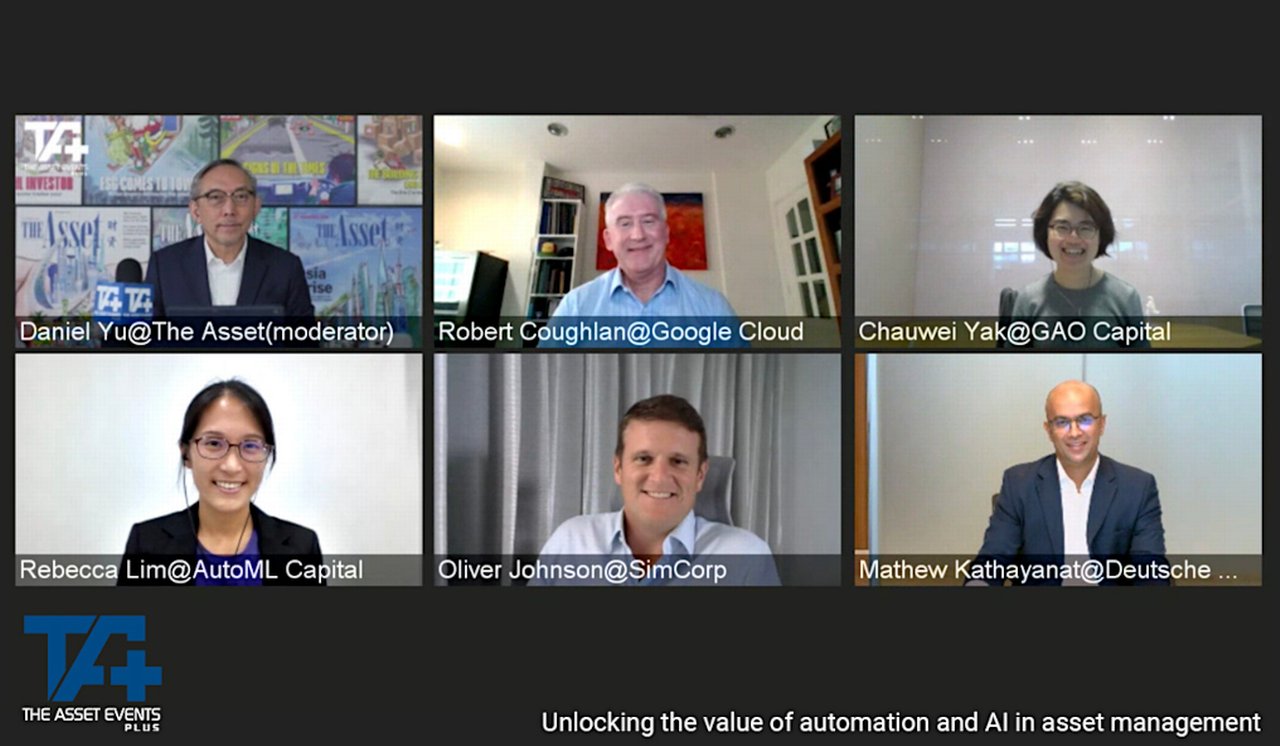
Pictured left to right: Mathew Kathayanat, Deutsche Bank, Daniel Yu, The Asset, Oliver Johnson, SimCorp, Robert Coughlan, Google Cloud, Rebecca Lim, AutoML Capital, Chauwei Yak, GAO Capital
Streamlining operational efficiency
Recent ‘black swan’ events, such as the Covid-19 pandemic, have accelerated asset managers’ plans to address key areas where technology could help increase the efficiency of operational processes, boost portfolio performance and improve risk management. The impact can already be seen throughout the industry.
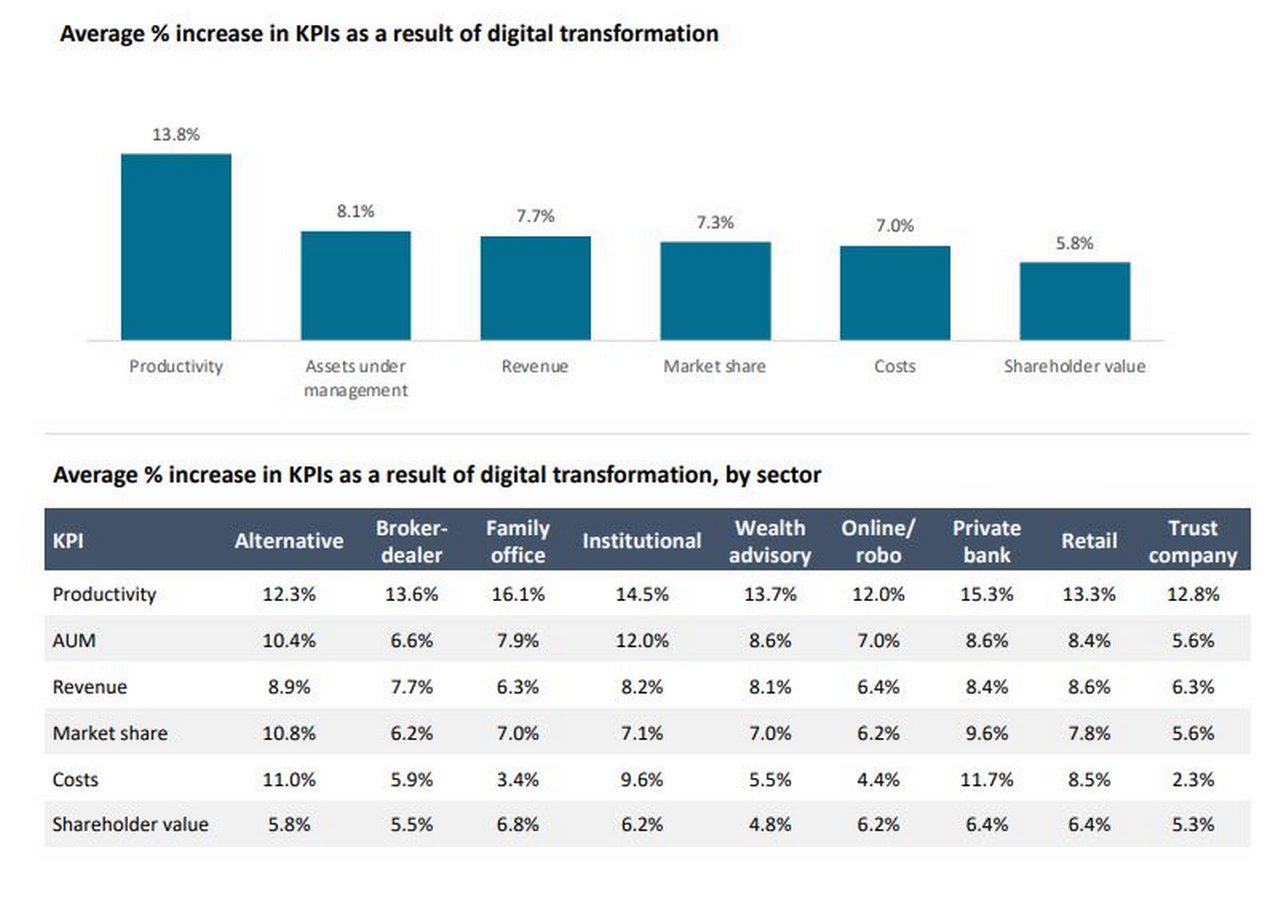
Figure 1: Digital transformation supercharges performance across multiple KPIs
Source: ESI Thoughtlab: Wealth and asset management 4.0
According to Wealth and Asset Management 4.0, a study from ESI ThoughtLab supported by Deloitte and other sponsors, that explored how Covid-19 is changing investor perspectives and service provider strategies, not only are asset management firms embracing digital transformation and automation, but these moves have also already increased their productivity by 13.8%, their assets under management (AUM) by 8.1%, and their revenue by 7.7%. See Figure 11 above.
This movement towards automation and AI started with the race to increase back-end operational efficiency. Triggered by what Kathayanat described as “the industry’s ‘Road to Damascus’ moment,” which saw “people working from home using legacy systems, while still having to meet client, market and reporting deadlines.” Unsurprisingly, it took little time for asset managers to change their approach – and there is now a growing focus on automating workflows using cloud and AI technologies.
“With the cloud, we can integrate our clients' complete corporate actions, without any capacity limits”
Custodian banks have been working to support this process too – making data available where needed to facilitate quicker and more efficient workflows. For Kathayanat, the cloud is a key enabler in this task. “Before the cloud, we could only transfer a certain amount of data attributes when exchanging corporate activities with buy-side customers using messaging API file feeds,” he explained. “With the cloud, we can integrate our clients' complete corporate actions without any capacity limits – giving our clients the flexibility they require.”
This also resonates with the fintech experience. Oliver Johnson, CCO of Singapore-based fintech SimCorp, highlighted the importance of operational efficiency as a driver of technology adoption for his asset management clients. “Most of our customers are telling us that the use cases fall into two buckets. It's either all about operational efficiency and how to get that increasing data load – how to sift through data and use it to drive down your costs – or it’s about how you use this data to ultimately make better investment decisions?”.
A webinar poll that asked attendees ‘Why automation and/or AI?’ aligned with Johnson’s summary. More than half of them indicated that they would like to use automation and/ or AI to ‘improve efficiency/ reduce risk, while more than one in four would use the technology for ‘better decision making’.
Regardless of the motivations, it is evident that there is a strong desire to implement these emerging technologies to revolutionise the traditional concepts of custody in asset management. “The future of custody is on the cloud,” suggested Kathayanat, who is hopeful that the future will solidify the ability to use automation and AI to import custody to the cloud, giving financial services the ability digitise the whole chain.
Using data to drive decision-making
The speed with which AI and ML can produce meaningful analytics from historically unstructured data is an exciting gain to many in the asset management industry. For firms seeking growth by outperforming the market, big data – and particularly so-called “alternative data” – can offer new strategies for generating alpha. Alternative data is information used by investors outside of the conventional sources offered by corporations and established industry data vendors. It can consist of anything from social media posts, satellite photos and news stories, through to granular cash-flow data –provided it also offers an insight into potential risk or investment performance.2
As Chauwei Yak, Founding Partner at GAO Capital outlined, AI and ML can automate and filter data and information from the daily news cycle. This can provide fast insight into market sentiment as well as saving time and resources for the asset managers. According to a London Stock Exchange Group survey in 2021 of 463 Data scientists, quants, model governance professionals and C-level executives working in financial services, more companies are now working to integrate unstructured data into their models, and fewer work exclusively with structured data (see Figure 2).3
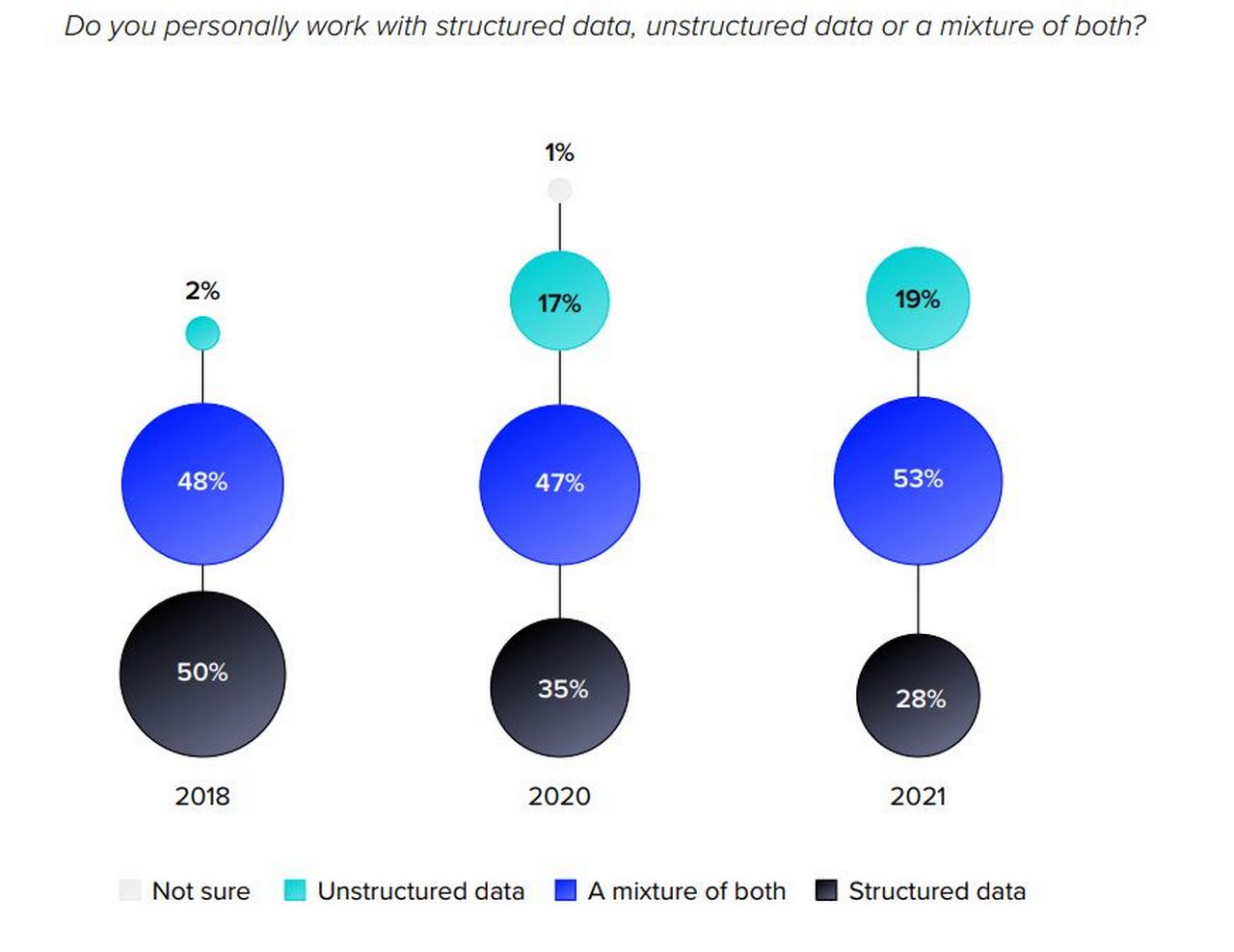
Figure 2: More companies now rely on structured and unstructured data
Source: The defining moment for data scientists (LSEG)
Digging deeper into ESG data
AI and ML also play a growing role in meeting demand for better insights into environmental, social and governance (ESG) performance. According to Bloomberg Intelligence, by 2025, ESG assets under management could account for more than a third of the anticipated worldwide total of US$140.5trn.4 China and India have made commitments to net zero, while Singapore and Hong Kong are now putting mandatory ESG into reporting regulations. The result for asset managers is increasing pressure to measure ESG criteria in their portfolios – AI and ML are proving important tools in the space, helping to automate the collection and interpretation of alternative data.
For example, AI and ML can create alternative datasets in ESG asset management. A programme taught to read the transcripts of a business's quarterly earnings calls could determine the tone and message of the CEO's words using natural-language processing to quickly identify those parts of the conversation where the CEO talks about ESG-related topics. Asset managers can then use those extracts to quickly drill down into the relevant information and determine how dedicated an organisation is about mitigating environmental risks and to what degree it is addressing the task in a structured and credible way.
Ethics, security and regulation
Yet, while enthusiasm for these technologies might be high among asset managers and their service providers, obstacles remain. Questions of ethics, security, data sovereignty and regulatory guardrails in the industry will require careful thought and negotiation as institutions look to innovate. Though the potential competitive advantage of using unstructured big data may seem seductive, the complexities of a data scientist’s day-to-day job is, “not as sexy as people think,” according to Rebecca Lim, Founding Partner at AutoML Capital. This, in part, is because data scientists must be constantly vigilant of AI ‘black box’ problems, AI ‘conceptual drift’ and ‘inferred bias.’
What did she mean by these concepts? An AI “black box” is where humans are no longer able to adequately explain or interpret the outputs of an AI model. This type of situation typically represents grounds for ethical and governance concerns. Lim noted the importance of “explainable AI” also known as “white box” construction. This means that the ML or AI process is broken down into, small, easily understandable steps.
Similarly, conceptual drift, or model drift is where the relationship between two variables in the input data starts to change. For example, the relationship between two input variables might shift from multiplication to addition without this being labelled to the model; a scenario the Deutsche Bank white paper, Unleashing the power of AI in securities services explains. As a result, the accuracy of the model outputs will start to drift. This effect can be amplified by the model’s attempts to learn from the new inputs, potentially leading to strange outcomes if not managed correctly. To mitigate the risk of conceptual drift, data scientists must be proactive in supervising their AI and ML tools, identifying and addressing any apparent drifts, and ensuring the data being fed in is relevant and explainable.
The third challenge – inferred bias – refers to the potential for discriminatory conclusions to be drawn from statistical calculations and other attributes based on limited data. This is among the most prominent challenges, according to Kathayanat, who noted: “The area where we haven't yet got a complete handle as an industry is how do we prevent inherent biases in AI?”
“You need to keep humans in the loop”
France’s regulator Authorite des Marchés Financiers (AMF) claimed that algorithms might perpetuate prejudices and result in the financial exclusion of certain groups. For instance, models based on consumer behaviour historically work less effectively for younger customers with a limited history.5 While correcting bias in AI and ML remains a considerable challenge, it was suggested that one way to quickly avoid discriminatory bias – while adhering to most markets’ data privacy and sovereignty laws – is to “mask the data.” That is, to modify sensitive data so that it is rendered unusable to unauthorised intruders but remains useable by the asset manager’s software or authorised workers.
The modern-day workflow
While the panelists reflected that the need to supervise, monitor and question the underlying processes of AI and ML technology will continue in the years to come, there is little debate as to whether or not the enterprise is worthwhile.
In the years ahead, ethical, regulatory, and social responsibility will remain at the core of the AI and ML asset management decision-making process – across portfolio, strategic and regulatory levels. “You need to keep humans in the loop,” concluded Coughlan. “AI is not a silver bullet, it’s a modern-day workflow.”
Summary of key points
- Digital transformation and automation are increasing asset management productivity and assets under management (AUM) levels
- More financial services firms rely on structured and unstructured data for market analysis
- AI and ML are also playing an increasing role in meeting demand for better insights into environmental, social and governance (ESG) performance
- However, model drift remains a risk and data scientists should be proactive in supervising their AI and ML tools, identifying and addressing any apparent drifts
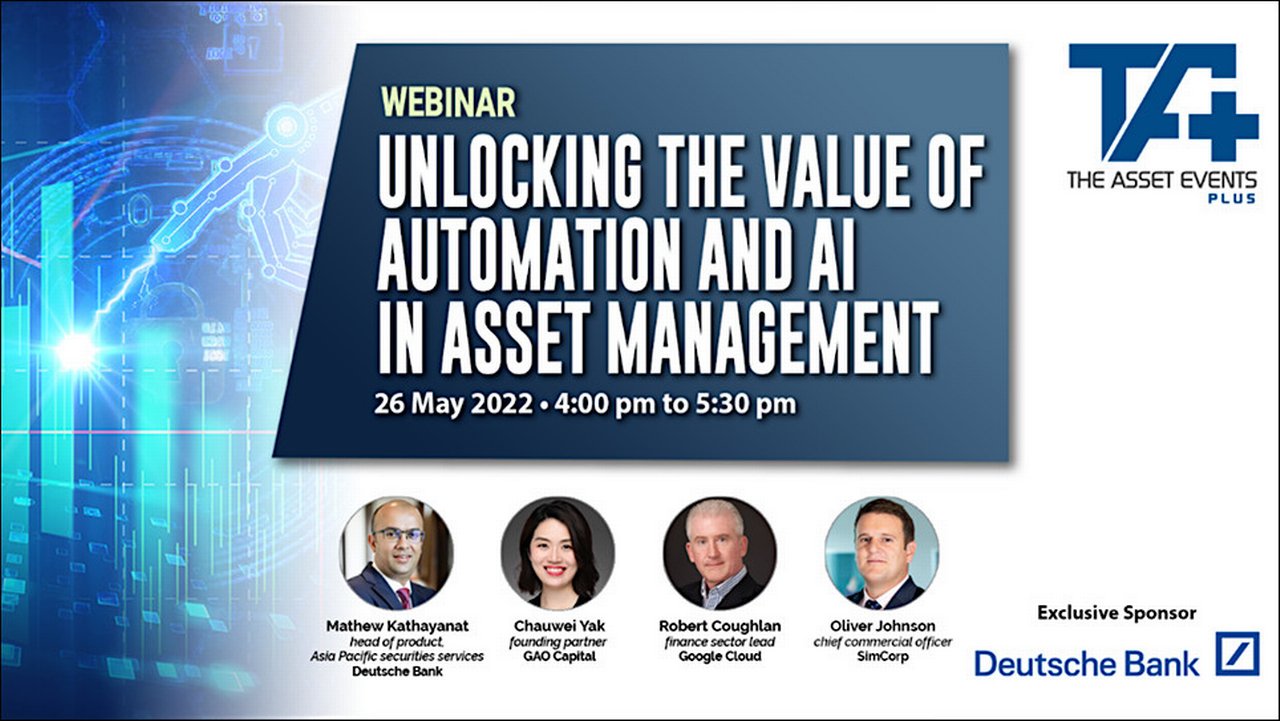
The webinar, ‘How will AI, ML, cloud services and automation shape the future of asset management?’ hosted by The Asset Magazine and sponsored by Deutsche Bank took place on 26 May 2022
Securities services solutions Explore more
Find out more about our Securities services solutions
Stay up-to-date with
Sign-up flow newsbites
Choose your preferred banking topics and we will send you updated emails based on your selection
Sign-up Sign-upSubscribe Subscribe to our magazine
flow magazine is published published annually and can be read online and delivered to your door in print
YOU MIGHT BE INTERESTED IN
SECURITIES SERVICES
Managing evolving networks Managing evolving networks
Network managers gathered at The Network Forum in London against a backdrop of regulatory reform, trade settlement compression momentum, digitalisation of assets and processes – and joy to be reconvening at a physical event. flow’s Clarissa Dann reports
CORPORATE BANK SOLUTIONS
The triple revolution in securities post-trade The triple revolution in securities post-trade
Digital assets custodial capabilities are under scrutiny and digital ecosystems such as decentralised finance (DeFi) and stablecoins in the cryptocurrency space have expanded the post-trade possibilities. Our new flow special white paper explains the implications for post-trade securities services
SECURITIES SERVICES, TECHNOLOGY
Unleashing the potential of AI in securities services Unleashing the potential of AI in securities services
Artificial intelligence (AI) is fast becoming an essential tool for the post-trade securities services and custody industry. As an increasing number of participants look to deploy the emerging technology to improve a wide range of processes, this Guide provides an introduction to AI in the field, including a rundown of use case examples, insight into important governance considerations and a series of key recommendations to ensure best practice is followed